AI and information retrieval
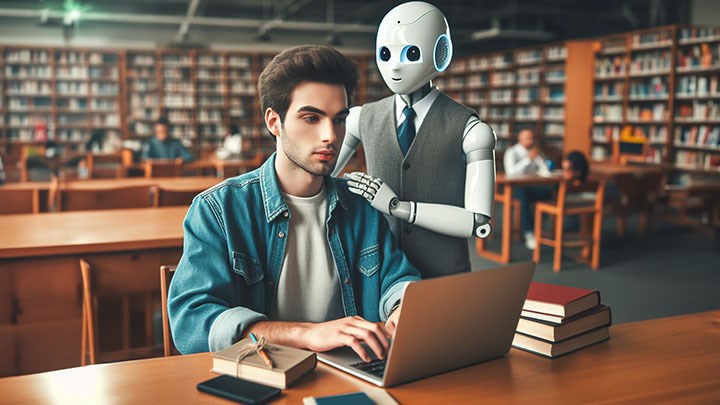
AI-genererated image from Bing Image Creator.
AI tools can be used to support and prepare information retrieval in several different ways. You can for instance use AI tools to find ideas for your thesis, suggested search terms, and articles.
Generative AI, ChatGPT and information retrieval
Tools based on generative AI such as ChatGPT may help you to obtain a quick overview of a topic. You can get help with the terminology used in the subject area, ask for summaries from different perspectives, and test your ideas.
Generative AI like ChatGPT is largely based on probability. If a sentence starts like this, how should it continue? These tools are usually unable to provide sources for their information because they do not search for sources. When they do indicate sources these may be completely fabricated.
When you are looking for scholarly articles as part of your studies, it is important to know that information is accurate and its source can be traced, and therefore it is better to use a database.
Information retrieval tools
Several tools use AI or other methods to help you to find similar material when you have already found some articles to start with. These tools may use citation analysis and semantic similarity to suggest additional articles. Some tools also use AI to interpret questions asked in natural language.
In citation analysis, further articles are suggested if they use the same source/s cited by the article you found.
In semantic searches, a language model is used to attempt to understand the meaning of a document or research question and to match it with similar content that does not necessarily use the same formulations. Instead of retrieving the results as a list of hits, some services present their results graphically and you can click your way to more related material.
Some tools use a technique called Retrieval Augmented Generation (RAG) to take advantage of language modelling and generative AI in information retrieval. The idea behind RAG is to use generative AI for understanding language but also to retrieve factual content from an external source. When searching, this could mean that a question is asked and answered in natural language while a background search is done in an academic database. The language model transforms the question into a query that the database understands and uses the articles found as context when answering the original question.
AI technology has already been incorporated into many of the more traditional databases the library subscribes to and several database providers plan to increase the use of AI in their services. AI can be used to extend search results, but also to rank results according to relevance or in some databases to generate summaries.